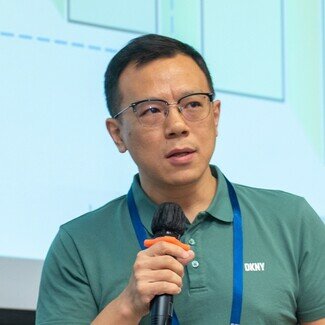
Prof. TIAN, Liang, 田亮教授
Associate Head
Associate Professor
B.Sc., Ph.D., Nanjing University of Aeronautics and Astronautics, Nanjing, China
Postdoctoral, Harvard Medical School, Boston, USA
Postdoctoral, Brigham and Women’s Hospital, Boston, USA
Current Research Interests
AI for Complex Systems
Dr. Tian’s group is dedicated to pioneering interdisciplinary research across a range of complex systems, where traditional analytic approaches falter due to a vast number of degrees of freedom with intricate interactions and structure involved. Our approach integrates principles and tools from statistical physics, network science, systems biology, and cutting-edge AI to develop a comprehensive understanding of these systems. At the core of our methodology is the identification and extraction of relevant statistics, dimensions, and features through advanced big-data mining and AI techniques. This allows us to construct statistical physics models and perform simulations that not only describe these systems accurately but also provide interpretative insights that are critical for further analysis and prediction. Our expertise uniquely positions us to reveal and understand the emergent properties and organizational principles of these systems, as well as the complex interplay between their structures and functions. Through this innovative research framework, Dr. Tian’s group aims to generate transformative insights and applications that will advance our understanding of complex systems in nature and technology.
Research Topics and Projects
(1) Human Microbiome and its Impact on Health and Disease
(2) AI for Traditional Chinese Medicine and Drug Discovery
(3) Complex Multimodal Brain Data and Computational Neuroscience
(4) Disease Transmission Modeling and Control
(5) Complex network and Nonlinear Systems: Structure and Dynamics
(6) Biological Big-data, Machine Learning, and Bioinformatics
Recent Selected Publications
- Xin Xiong, Yerong Liu, Dandan Pu, Zhu Yang, Zedong Bi, Liang Tian#, Xuefei Li#, DeSide: A unified deep learning approach for cellular deconvolution of tumor microenvironment, PNAS, 121, e2407096121 (2024) (# Corresponding)
- Zedong Bi#, Haoran Li, Liang Tian#, Top-Down Generation of Low-Resolution Representations Improves Visual Perception and Imagination, Neural Networks, 171, 440 (2024) (# Corresponding)
- Mi Feng, Liang Tian#, Ying-Cheng Lai, Changsong Zhou#, Validity of Markovian modeling for transient memory-dependent epidemic dynamics, Communications Physics, 7, 86 (2024) (# Corresponding)
- Liang Tian, Xuefei Li, Fei Qi, Qianyuan Tang, Viola Tang, Jiang Liu, Zhiyuan Li, Xingye Cheng, Xuanxuan Li, Yingchen Shi, Haiguang Liu, Leihan Tang, Harnessing peak transmission around symptom onset for non-pharmaceutical intervention and containment of the COVID-19 pandemic, Nature Communications, 12, 1147 (2021)
- Liang Tian, Xu-Wen Wang, Ang-Kun Wu, Yuhang Fan, Jonathan Friedman, Amber Dahlin, Matthew K. Waldor, George M. Weinstock, Scott T. Weiss, Yang-Yu Liu, Deciphering Functional Redundancy in the Human Microbiome, Nature Communications, 11, 6217 (2020)
- Liang Tian, Amir Bashan, Da-Ning Shi, and Yang-Yu Liu, Articulation points in complex networks, Nature Communications, 8, 14223 (2017)
- Linda Zhong, Liang Tian, Chester Yan Jie Ng, Choryin Leung, Xian Yang, Ching Liong, Haiyong Chen, Rowena Wong, Bacon FL. Ng, Z.X. Lin, Y.B. Feng, Z.X. Bian, Persistent clinical symptoms and their association with CM syndromes in post-COVID-19 rehabilitation patients in Hong Kong, Heliyon, e19410 (2023)
- Qingnan Rong, Jun Zhang, Xiaoqian Sun, Sebastian Wandelt, Massimiliano Zanin, and Liang Tian, Optimal Cost-based Strengthening of Complex Networks, IEEE Transactions on Network Science and Engineering, 9, 1117 (2022)
- Ang-Kun Wu, Liang Tian, Bruno Coelho Coutinho, Yasser Omar, Yang-Yu Liu, Structural vulnerability of quantum networks, Physical Review A, 101, 052315 (2020)
- Yandong Xiao, Chuliang Song, Liang Tian, Yang-Yu Liu, Accelerating the emergence of order in swarming systems, Advances in Complex Systems, 1950015 (2020)
- 陈沫先, 韦中, 田亮, 谭扬, 黄建东, 戴磊, 合成微生物群落的构建与应用, 科学通报, 66, 273-283 (2020).